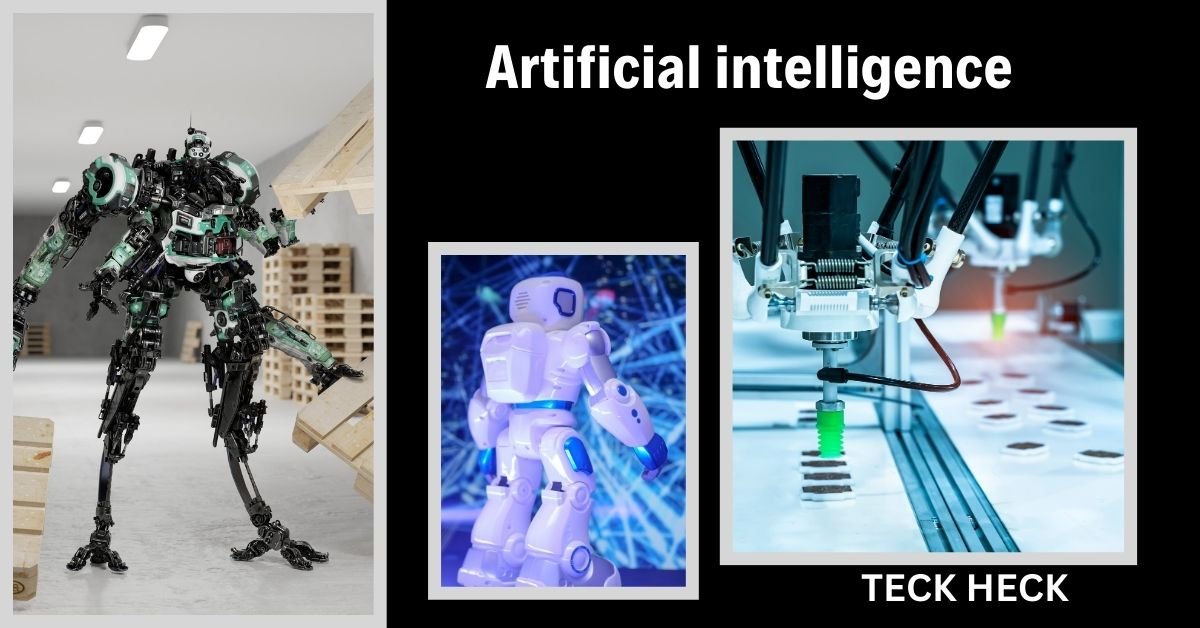
The most popular buzzword right now is artificial intelligence (AI), and practically all of the big businesses are incorporating AI into their products or services. Regretfully, despite the fact that the term seems to need a simple and straightforward meaning, it doesn’t. Some marketing departments are promoting what researchers refer to as a slight advancement in machine learning as a major step toward artificial intelligence (we will get to that one too).
As sci-fi author Ted Chiang put it in a profile in the Financial Times, artificial intelligence was “a terrible choice of words in 1954.” And I think there is a lot of truth to it, having written about AI advancements for the past ten years. It is difficult to have a meaningful conversation on AI without first defining the terms and meanings used because they are so vague.
Artificial intelligence, in its broadest definition, is the ability of a machine to learn, make decisions, and act, even in the face of a circumstance it has never encountered before.
Many people intuitively believe that, in the most limited sci-fi meaning, artificial intelligence (AI) refers to computers and robots that possess human or superhuman intelligence and enough personality to function as characters rather than merely story devices. Although the computer in Star Trek is merely an enhanced version of Microsoft Clippy, Data is an artificial intelligence. This definition is not met by any AI in the present era.
Simply put, a non-AI computer program is designed to consistently perform the same task in the same manner. Consider a robot that bends a little piece of wire to create paper clips. It consistently makes the same three bends with the few inches of wire. It will continue to bend wire into paper clips as long as it is supplied with wire. But if you give it a piece of dry spaghetti, it will simply break it. It can only bend a strip of wire; it is incapable of anything else. It can not adjust to a new scenario on its own, but it could be reprogrammed.
Conversely, AIs may learn and tackle increasingly dynamic and complicated situations, including ones they have not seen before. Not a single business is attempting to teach a computer to navigate every intersection on every road in the United States in the race to develop a driverless automobile. Rather, they are trying to develop computer programs that can employ a range of sensors to sense their environment and respond appropriately to real-world circumstances, even if they have never encountered them before. Although a fully autonomous vehicle is still a ways off, it is evident that they cannot be developed in the same manner as standard computer programs. The coders simply cannot take into consideration every case.
Naturally, you would wonder if a car that drives itself would be intelligent. For most definitions of intelligence, it is unquestionably more thoughtful than a robotic vacuum cleaner, but the answer is probably a big maybe. Building an artificial general intelligence (AGI) or strong AI—an AI with human-like intelligence that can learn new tasks, communicate, comprehend instructions in various formats, and realize all of our sci-fi fantasies—would be the true triumph of artificial intelligence. This is still a long way off, to reiterate.
The current state of artificial intelligence (AI) is frequently referred to as weak AI, narrow AI, or artificial narrow intelligence (ANI): AIs that are educated to carry out particular tasks but are not all-inclusive. Some fairly amazing uses are still made possible by this. Despite being rather basic ANIs, Alexa from Amazon and Siri from Apple are both capable of handling a large number of requests.
Given how common AI is right now, we will probably hear the word used frequently for meaningless purposes. Therefore, when you see a brand using the notion to market itself, be sure it is AI and not just a set of rules by doing some research. This leads me to the following point.
How does artificial intelligence operate?
The majority of AIs today rely on a procedure known as machine learning to create the intricate algorithms that make up their intelligence. Although many real-world applications of AI also heavily rely on other fields of AI research, such as robotics, computer vision, and natural language processing, machine learning remains the foundation for training and development.
A computer software is given a sizable training data set—the larger, the better—for machine learning. Let us say you wish to teach a computer to distinguish between various animals. Thousands of images of animals with text labels explaining them could make up your data set. The computer software might develop an algorithm—a set of rules, actually—for recognizing the various species by running through the entire training data set. The computer software would generate its own set of criteria rather than requiring a human to program one.
This implies that companies who have data to train AI on, such as consumer queries, will have the greatest success implementing AI.
While the details become much more intricate, the foundation of both GPT-3 and GPT-4 (Generative Pre-trained Transformer 3/4) and Stable Diffusion is structured training through machine learning. Nearly 500 billion “tokens”—roughly four characters of text—from books, news stories, and websites throughout the internet were used to train GPT-3, the GPT in ChatGPT. In contrast, Stable Diffusion made use of the 5.85 billion text-image pairs in the LAOIN-5B dataset.
The neural networks—complex, multi-layered, weighted algorithms fashioned after the human brain—that the GPT models and Stable Diffusion created from these training datasets enable them to forecast and produce new content based on the knowledge they have gained from their training data. ChatGPT responds to queries by predicting the next token to be sent via its neural network. Stable Diffusion transforms a set of random noise into an image that corresponds to the text when you tell it to do so using its neural network.
Technically, both of these neural networks are “deep learning algorithms.” Despite the fact that the terms are frequently used interchangeably, modern artificial intelligence relies on deep neural networks, which frequently consider millions or billions of parameters, whereas a neural network can theoretically be rather basic. Because the details of what they are doing are difficult to dissect, this leaves their activities unclear to end users. When it comes to prejudiced or otherwise unpleasant content, these AIs can be problematic because they are frequently black boxes that receive an input and produce an output.
Additionally, AIs can be trained in different ways. AlphaZero played millions of games against itself to learn how to play chess. At first, it was just aware of the win condition and the fundamental game rules. As it experimented with various tactics, it discovered what worked and what didn’t, and it even came up with some ideas that humans had not previously thought of.
Basics of AI: definitions and terminology
AI is currently capable of a vast array of amazing technological tasks, frequently by integrating many capabilities. These are a few of its main capabilities.
Learning by machine
Machine learning is the process by which computers (machines) extract knowledge from material they have been taught on and then use that knowledge to start learning new things. A large dataset is sent to the computer, which is educated by humans in a variety of methods before learning to adapt.
Deep learning
A “deep” component of machine learning is deep learning, which allows computers to function even more independently with less assistance from humans. A deep learning neural network is a sophisticated, multi-layered, weighted algorithm that is modeled after the human brain and is created using the enormous dataset that the computer is trained on. This implies that information (and other kinds of data) can be processed by deep learning algorithms in a highly sophisticated, human-like manner.
Based on their training data, generative AIs such as GPT and DALL·E 2 may produce new material from your inputs.
For instance, GPT-3 and GPT-4 were trained on an unfathomable amount of written material. It essentially includes hundreds of thousands of books, papers, and other publications in addition to the entirety of the public internet. This explains why they can comprehend your written prompts and engage in lengthy discussions about Shakespeare, the Oxford comma, and which Slack emojis are unsuitable for professional settings. Their training data has everything they need to know about them.
AIs are capable of much more with language than merely creating text. AIs can comprehend, categorize, evaluate, respond to, and even translate everyday human communication thanks to natural language processing, or NLP.
For instance, there are dozens of ways to word or frame the request to turn on the lights in a room. A machine that has a basic knowledge of English can react to certain keywords. (As an illustration, “Alexa, lights on.”) However, natural language processing (NLP) is what enables artificial intelligence to understand the more intricate expressions that individuals use in everyday conversation.